

Google’s DeepMind unit, the same division that created AlphaGo, an AI that outplayed the best Go player in the world, has created a neural network capable of rendering an accurate 3D environment from just a few still images, filling in the gaps with an AI form of perceptual intuition.
According to Google’s official DeepMind blog, the goal of its recent AI project is to make neural networks easier and simpler to train. Today’s most advanced AI-powered visual recognition systems are trained through the use of large datasets comprised of images that are human-annotated. This makes training a very tedious, lengthy, and expensive process, as every aspect of every object in each scene in the dataset has to be labeled by a person.
The DeepMind team’s new AI, dubbed the Generative Query Network (GQN) is designed to remove this dependency on human-annotated data, as the GQN is designed to infer a space’s three-dimensional layout and features despite being provided with only partial images of a space.
Similar to babies and animals, DeepMind’s GQN learns by making observations of the world around it. By doing so, DeepMind’s new AI learns about plausible scenes and their geometrical properties even without human labeling. The GQN is comprised of two parts — a representation network that produces a vector describing a scene and a generation network that “imagines” the scene from a previously unobserved viewpoint. So far, the results of DeepMind’s training for the AI have been encouraging, with the GQN being able to create representations of objects and rooms based on just a single image.
As noted by the DeepMind team, however, the training methods that have been used for the development of the GQN are still limited compared to traditional computer vision techniques. The AI creators, however, remain optimistic that as new sources of data become available and as improvements in hardware get introduced, the applications for the GQN framework could move over to higher-resolution images of real-world scenes. Ultimately, the DeepMind team believes that the GQN could be a useful system in technologies such as augmented reality and self-driving vehicles by giving them a form of perceptual intuition – extremely desirable for companies focused on autonomy, like Tesla.
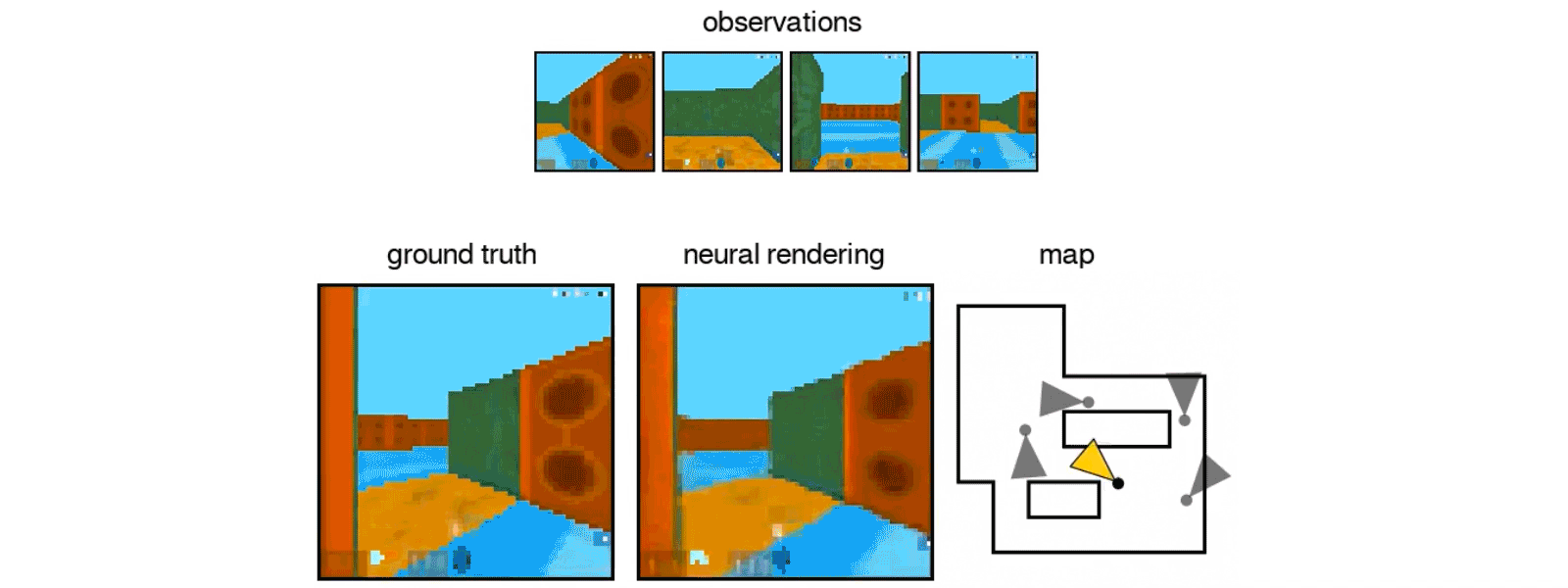
Google DeepMind’s GQN AI in action. [Credit: Google DeepMind]
In a talk at Train AI 2018 last May, Tesla’s head of AI Andrej Karpathy discussed the challenges involved in training the company’s Autopilot system. Tesla trains Autopilot by feeding the system with massive data sets from the company’s fleet of vehicles. This data is collected through means such as Shadow Mode, which allows the company to gather statistical data to show false positives and false negatives of Autopilot software.
During his talk, Karpathy discussed how features such as blinker detection become challenging for Tesla’s neural network to learn, considering that vehicles on the road have their turn signals off most of the time and blinkers have a high variability from one car brand to another. Karpathy also discussed how Tesla has transitioned a huge portion of its AI team to labeling roles, doing the human annotation that Google DeepMind explicitly wants to avoid with the GQN.
Musk also mentioned that its upcoming all-electric supercar — the next-generation Tesla Roadster — would feature an “Augmented Mode” that would enhance drivers’ capability to operate the high-performance vehicle. With Tesla’s flagship supercar seemingly set on embracing AR technology, the emergence of new techniques for training AI such as Google DeepMind’s GQN would be a perfect fit for the next generation of vehicles about to enter the automotive market.

Interested in solar? Get a solar cost estimate and find out how much a solar system would cost for your home or business.